Updated: April 4, 2025
The lowest-performing sector was the verdict given to utilities in the UK by the research of Utility and PayPal.
Back in 2023, the UK Customer Satisfaction Index (UKCSI) signalled a significant downward shift in customer satisfaction within the utilities sector, with scores falling from 74.1 to 69.5.
This significant drop placed the utilities sector at the bottom of the satisfaction rankings, 7.1 points below the national average across all sectors.
In detail:
- The energy sector experienced a notable decrease in satisfaction, primarily due to significant price increases, with a decline of 5.3 points bringing it down to 67.9.
- The water sector, although performing slightly better, also saw a decrease in satisfaction by 3.5 points to 72.6, influenced by incidents related to storm overflows.
Jo Causon, CEO of the Institute of Customer Service (ICS), suggested that utility companies should prioritise digital enhancements to boost customer satisfaction.
At MaxBill, we realise the importance of customer loyalty in the utility business. That’s why we came up with a customer churn prediction model. It identifies the customers about to leave before they actually leave and provides recommendations to make them stay.
Keep on reading as we dive into the ins and outs of such models and help you understand if they will work for your specific business case. and outs of such models and help you understand if they will work for your specific business case.
Key Takeaways
- Churn prediction models is about gaining a strategic capability for your organisation to protect revenue, boost internal efficiency and morale, and create the conditions for sustainable growth.
- ML-driven models might be built on logistic regression, decision trees, neural networks or ensemble methods to gain visibility on why customers leave, who exactly and where (location) and what to do about it while processing data responsibly.
- Customer churn prediction models is a way to go for utility providers aiming at reducing preventable revenue loss, removing frustration in call centers and processes sabotaging growth, and leveling up customer interactions in general.
- MaxBill churn prediction model comes as a pre-existing one but factors in the distinct nature of each business, catering to the unique needs of every company.
- MaxBill’s model is built upon an impressive array of over 50 parameters, meticulously selected for their impact on customer churn prediction (CCP).
- Based on predefined parameters, the model identifies contracts with a high likelihood of churn.
- Built-in ‘What-if simulation” capacity allows anticipating potential scenarios of what can possibly work to make customers not only stay but even make them more loyal.
- Hyperautomation is key here: as soon as the model detects a customer or group at risk of churn, the system automatically triggers the preferred churn prevention actions, eliminating the need for human intervention.
Customer churn prediction and ML: what utility CTOs should know?
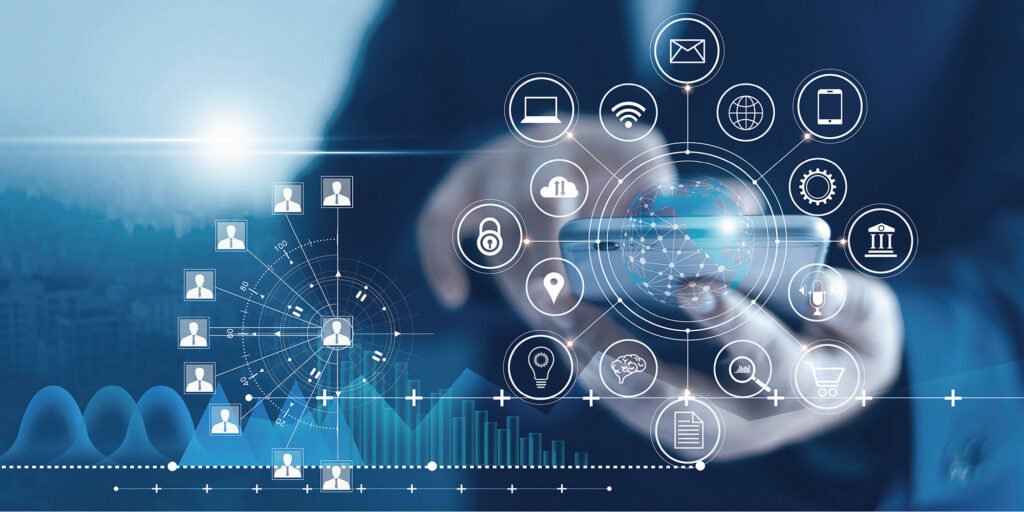
The models are not about exercising data science, it’s gaining a strategic capability for your organisation.
At their core, the ML-driven models analyze historical data points and current patterns to predict customer churn, allowing your teams to act early—whether by resolving service pain points, tailoring offers, or improving communication.
Without diving into tech terms, let’s outline the key aspects of machine learning predictive models and how they help customer-facing teams.
Logistic regression tells why customers leave
Logistic regression is a foundational algorithm that estimates the likelihood of a binary outcome—in this case, whether a customer will churn or stay. It’s ideal when you need both predictive power and clear explanations behind each prediction.
Utility companies often collect structured customer data over long customer lifecycles, which logistic regression can utilize effectively. It offers visibility into “why” churn happens—whether due to billing issues, service interruptions, or unresolved complaints—making it an actionable tool for operations teams.
Decision trees make customer behavior clearer
In the whole process of customer churn prediction, decision trees break down decisions into a tree-like structure of questions, allowing you to trace the exact paths that lead to churn.
With diverse customer profiles and usage behaviors, decision trees offer an intuitive way to spot at-risk groups and act accordingly. For instance, customers in a particular region who faced repeated outages and contacted support multiple times may appear as a high-churn segment, triggering a local intervention plan.
Neural networks provide more nuanced customer feedback
Neural networks model complex, nonlinear relationships in data—ideal for when customer churn patterns are influenced by multiple overlapping behaviors.
As utilities expand their digital footprints—smart meters, mobile apps, online self-service—customer interactions generate vast, nuanced data points. Neural networks are well-suited for mining these signals for churn risk. They can outperform simpler models in prediction accuracy, especially when customers behave in less predictable ways.
Ensemble Methods increase effectiveness and intelligence
Ensemble models combine customer churn predictions from multiple algorithms to increase accuracy and robustness.
In real-world utility data, no single churn prediction model always wins. Some patterns are linear, others nonlinear; some are clear-cut, others buried in noise. Ensemble methods embrace this complexity and deliver more stable, generalizable predictions across customer groups and service types.
High-quality, integrated customer data as key to success
Even the most sophisticated models are only as good as the data feeding them. CIOs must prioritize data integration across billing systems, CRM platforms, outage records, call centre logs, and digital interactions.
Key customer data types include transactional data, service interaction data, behavioral signals, and some external signals, such as social media sentiment, regional trends, weather patterns.
With regulations like GDPR and growing scrutiny over AI use, utilities must ensure customer information is anonymized where possible, stored securely, and used transparently. Responsible data practices are not just legal necessities—they also build customer trust.
Why to predict customer churn?
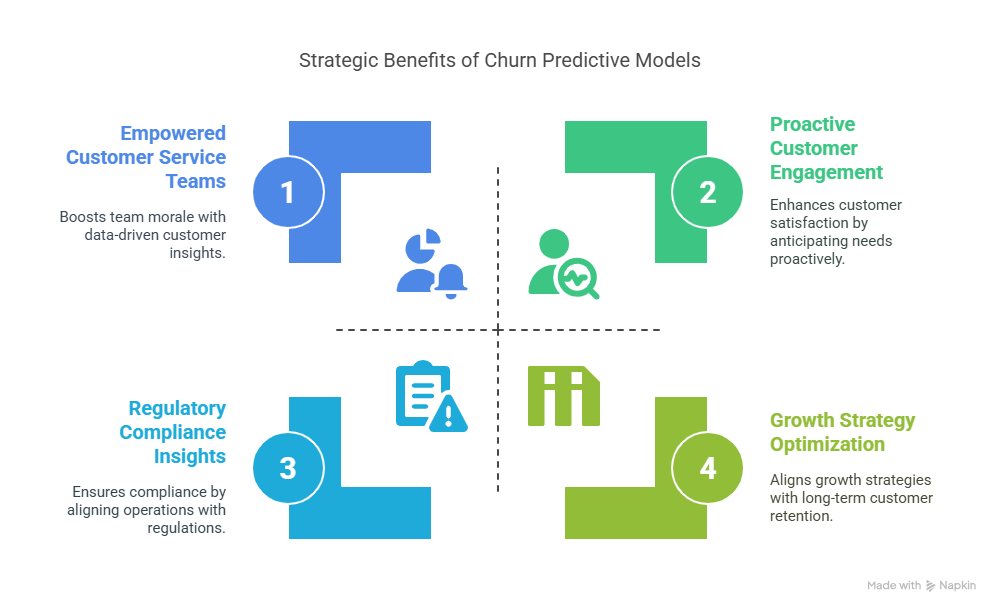
The priorities for utility business leaders are always crystal clear: protect and stabilize revenue, boost internal efficiency and morale, and create the conditions for sustainable growth. We’ll break down the outcomes the machine learning model brings to energy and utility businesses.
Reducing preventable revenue loss
In utility businesses, customers leaving—whether due to dissatisfaction, better offers from competitors, or changing usage patterns—directly translates into a drop in monthly recurring revenue.
However, what’s often overlooked is that churn also includes partial disengagement: for example, when a customer moves to a lower-tier plan or drastically reduces their usage.
Again, by identifying at-risk customers before they fully disengage, predictive models give your team the chance to intervene early—through proactive outreach, customized service adjustments, or loyalty incentives.
This proactive retention strategy not only anticipates customer churn and prevents complete customer loss but can also reduce the incidence of downgraded services, helping stabilize cash flow and long-term revenue performance.
For utility CTOs, this means fewer surprises in revenue forecasts and a more resilient business model in volatile markets.
Equipping customer-facing teams with the right tools or data
Customer churn doesn’t just affect the bottom line. High client loss can frustrate frontline employees, especially those in call centers, billing, and customer success, who often feel the pressure of trying to retain dissatisfied users without the right tools or data. Over time, this can lead to burnout, higher turnover, and declining morale.
With the model, rather than operating reactively, they gain early signals about which customers need attention and why—allowing them to resolve issues more efficiently and with greater confidence.
For CTOs, enabling this shift means providing access to actionable insights through dashboards, workflows, or alerts that integrate directly into operational systems. As a result, the same resources can deliver higher impact—reducing the cost of churn mitigation while boosting team performance and satisfaction.
Removing bottlenecks to growth trajectories
The quiet outflow of existing customers can undermine the process of gaining new customers and slow down or even reverse growth strategies, in general.
A well-functioning churn prediction model allows your organization to predict customer churn more accurately, plan smarter customer experience strategies, and retain the users most critical to long-term value.
In the long run, this fosters healthier customer cohorts, reduces the pressure on acquisition costs, and creates the conditions for scaling more predictably.
For CTOs, this means being able to support strategic business objectives with real data, ensuring that IT, analytics, and operations are aligned in creating long-term customer value—not just reacting to short-term losses.
Ensuring the balance between regulations and customer expectations
EU Regulations from one side and customer expectations from another one (if Amazon can deliver great customer experience, why can’t utilities?) oblige businesses to pay a particular attention at how they interact with their clients.
This is where a machine learning model is a way to go. Not only does it predict disengagement and often suggests pro-active measures to satisfy customer needs but also creates a win-win situation for both – business and clients.
Additionally, the oversight from consumer watchdogs, such as Ofgem, poses a risk of rating downgrades and company’s reputational damage, highlighting the critical role of exceptional customer service.
What is the MaxBill churn prediction model?
MaxBill’s model was initially crafted for the telecom sector. The model was initially crafted for the telecom sector. Further, it evolved into addressing the challenges posed by stiff competition and the trend of customers switching for better deals.
Today, MaxBill’s churn prediction model is versatile and serves multi-service providers, including telecommunications, internet, TV, and multi-utility companies. It comes as a stand-alone solution, besides MaxBill’s modern utility billing software.
MaxBill’s churn prediction model is not an out-of-the-box solution. It’s a testament to how MaxBill provides machine learning as an additional service, catering to the unique needs and requirements of each business.
This approach recognises that the model should align with the specific use cases of the business, rather than expecting businesses to adapt to pre-existing churn models. MaxBill can factor in the distinct nature of each business, leveraging their access to data to customise the model.
MaxBill’s Churn Prediction Model in Action
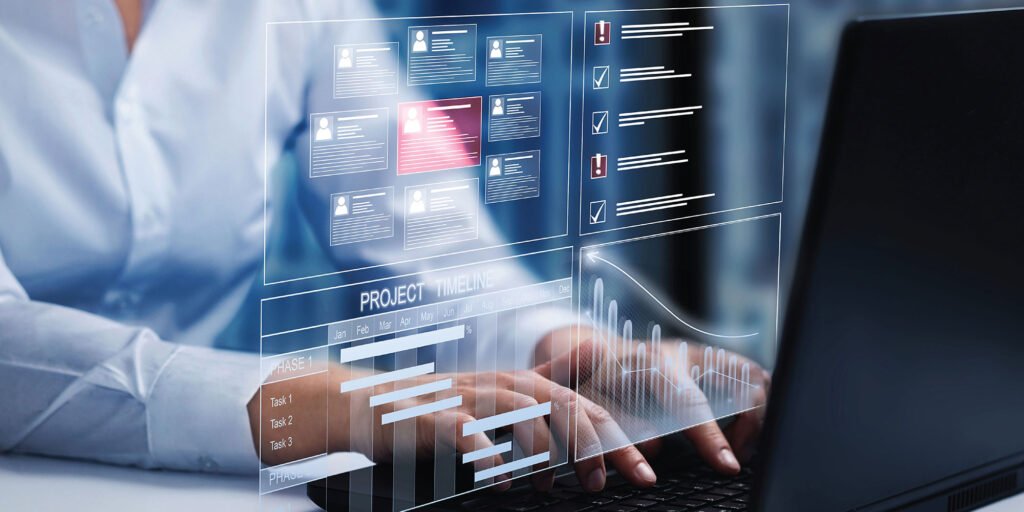
MaxBill’s model is built upon an impressive array of over 50 parameters, meticulously selected for their impact on customer churn prediction (CCP). The method features:
Seamless Integration: the churn model for utilities seamlessly integrates into a client’s system through a responsive API. This integration allows for the analysis of data from any period in time, ensuring businesses have access to the most relevant insights.
High Accuracy: Churn modelling boasts high accuracy in predicting customer attrition thanks to utilising XGBoost and Flask, a powerful technology stack.
Local Interpretability: Predicting churn incorporates the Shapley value mechanism, enabling local interpretability. It pinpoints which features have the most significant impact on churn and which have a lesser influence.
Customisable Parameters: The churn model offers a range of parameters that can be tailored to suit the specific needs of the industry and the client. Flexibility is key since the model’s efficacy hinges on its adaptability.
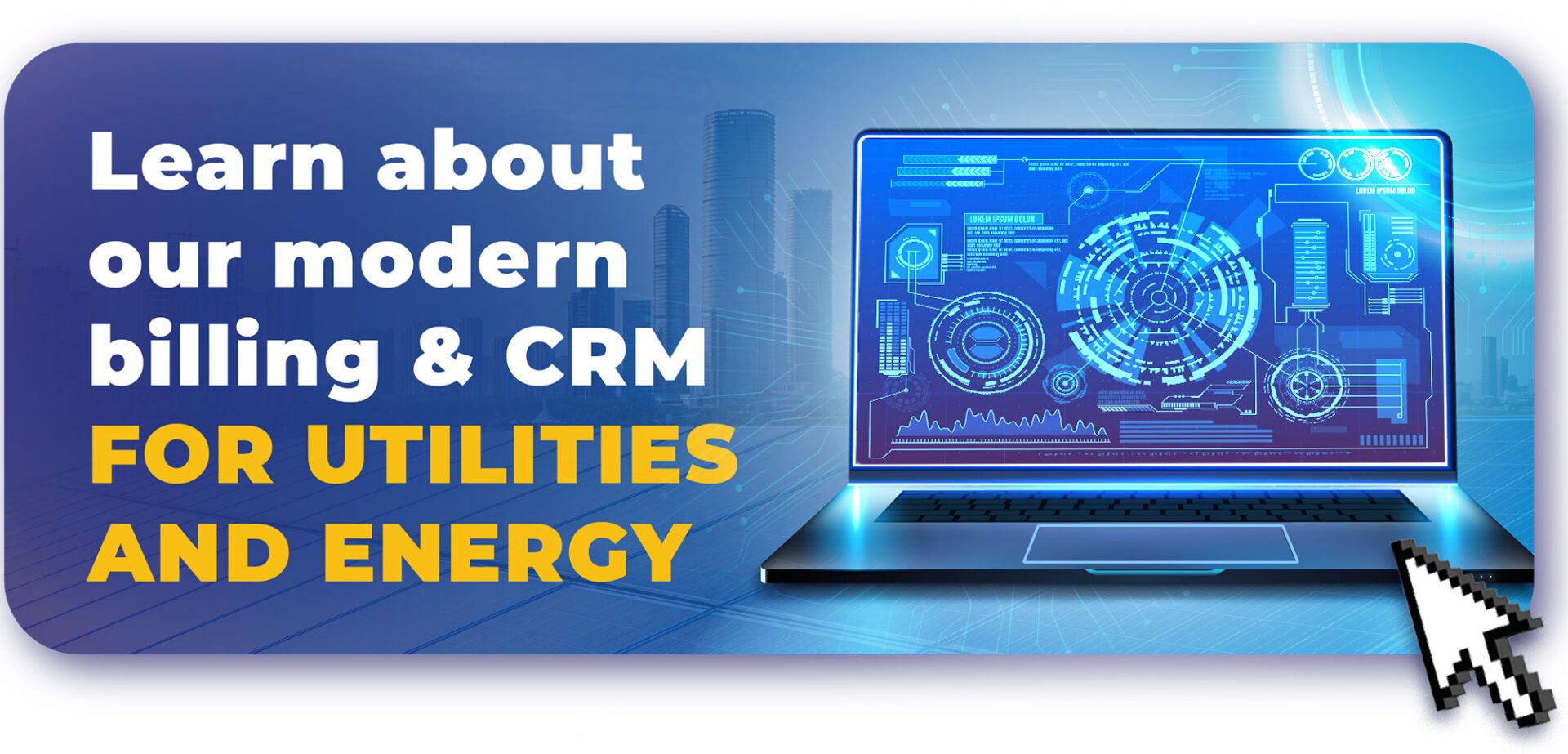
Anticipating the future: ‘What-if’ scenarios in predicting customer churn
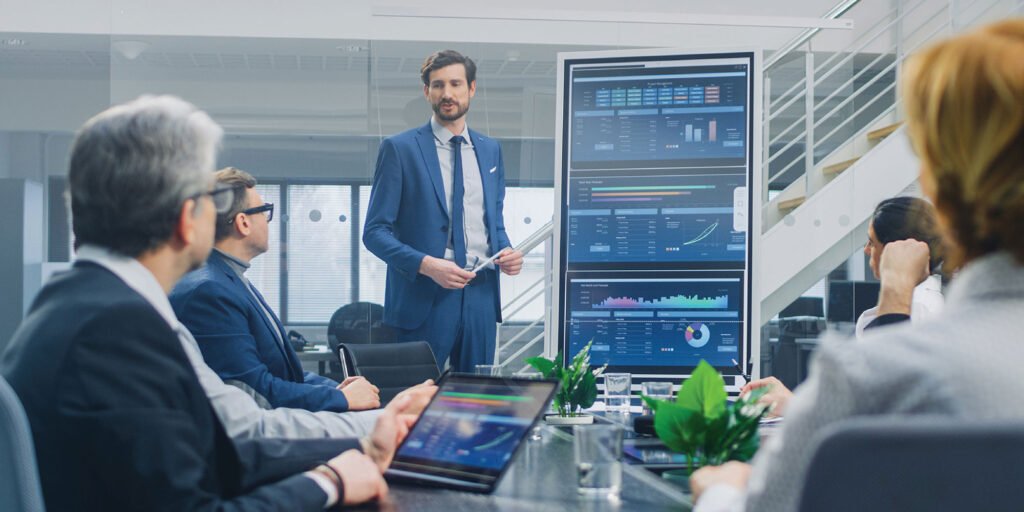
One of the customer churn modelling’s compelling features is its ability to simulate ‘what-if’ scenarios. The model is trained on specific churned customer data of specific businesses, each having particular parameters.
With the system, companies can explore how changes in parameters affect attrition. For example, a customer changing to a basic internet tariff with a slower speed reduced the probability of churn from 53% to 6%.
It’s pivotal to mention the role of automation in proactive churn prediction. Once the system detects a customer or group at risk of churn, automated churn prevention actions can be initiated, eliminating the need for human intervention.
Under the hood: how the predictive churn model operates
Understanding how the model works is essential for businesses looking to harness its power to retain customers and reduce churn. Let’s delve into the core functions of the churn model, which are instrumental in achieving these goals:
Selecting Contracts with High Attrition Probability: Based on predefined parameters, the model identifies contracts with a high likelihood of churn.
Data Analysis: It draws its insights from historical data, typically spanning 12 months and evaluated over 3 months.
Parameter Determination: The approach allows determining the parameters that significantly influence the possibility of customer churn.
Recommendations and Customer Retention: The system behind it not only identifies at-risk customers but also recommends changes to retain them. It does really level up customer interactions with utility providers.
A Glimpse into the MaxBill Churn Prediction Model for Energy & Utilities
Strategic implementation: customer retention strategies
Understanding how to effectively deploy a churn prediction model is paramount. Without a clear grasp of how to predict customer churn, even the most sophisticated models can fall short. Let’s explore how our model can be strategically leveraged:
Per-customer insights to predict customer churn
When seamlessly integrated with the company’s CRM, the churn prediction engine offers a valuable tool for customer service representatives (CSRs). It provides these frontline agents with deep insights into each client’s likelihood of ending their relationship with our company. Armed with this intelligence, our CSRs can take proactive measures, tailoring their interactions to reduce churn risks and foster lasting client relationships.
Bulk reporting
The customer churn prediction model generates comprehensive reports on specified dates, pinpointing the list of customers within the ‘at-risk’ category. This data serves as the foundation for targeted customer retention marketing campaigns. This enables organisations to not only mitigate churn but also engage customers with their services and enhance brand loyalty.
Interactive management
Our model’s capabilities extend beyond mere identification to predict customer churn. It is a dynamic, interactive tool that empowers management to proactively influence customer retention.
This tool helps implement retention strategies like new pricing offers, discounts, and special promotions. Then, it allows business analytics to assess the anticipated results, taking into account any newly introduced variables in the model.
This versatility is invaluable for crafting tailored offers for specific customer segments while striking the optimal balance between marketing ROI and customer churn, all while maintaining a low false-positive rate.
The icing on the cake for helping to predict customer churn is the automation built into our system. As soon as the model detects a customer or group at risk of churn, the system automatically triggers the preferred churn prevention actions, eliminating the need for human intervention. This ensures that an organisation is always one step ahead in retaining valued customers.
Related:
Proactive Path: How We Deliver a Predictive Model for Utility Churn Reduction
Utility Debt Prediction Model to Protect Revenue and Enhance Customer Retention
Utility Debt Management With Optimised Collection Systems
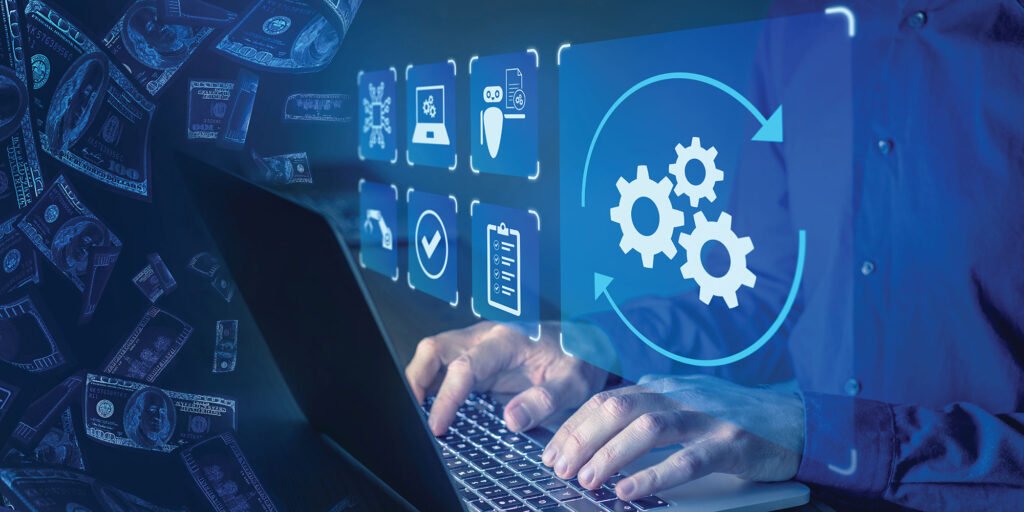
Are you willing to adopt MaxBill’s predictive model as a separate service or integrate it seamlessly with our utilities billing solution? Contact our experts today to explore how MaxBill can empower your utility organisation to thrive in the competitive market!
Request MaxBill’s webinar on innovative models!
Discover MaxBill’s webinar on how leveraging machine learning and AI can revolutionise utilities and energy management, enhancing operational efficiency, reducing customer churn, and ensuring revenue protection. Gain exclusive insights from industry experts into predictive modelling for optimised operations, tailored solutions, and real-world applications. This is a pivotal opportunity for C-level Utility executives to drive innovation, foster transformative business outcomes, and lead their organisations into a new era of technological advancement and customer satisfaction.